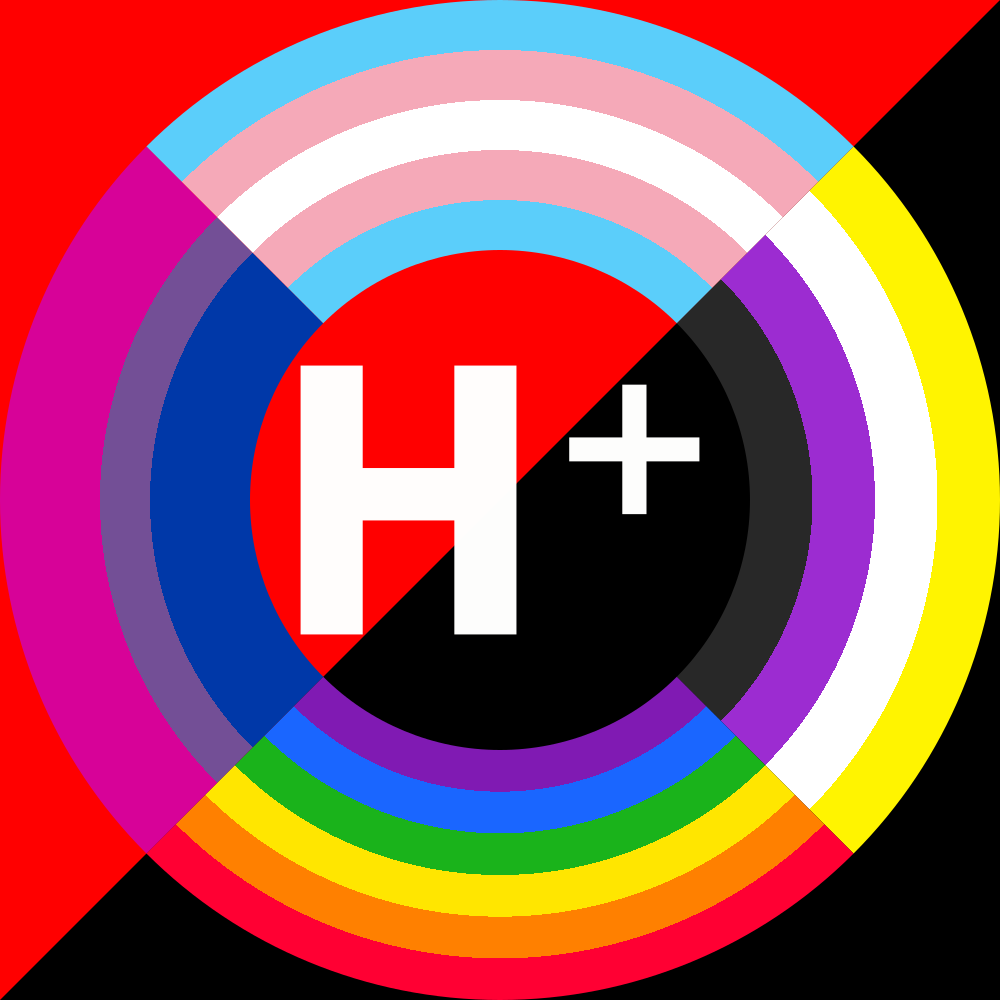
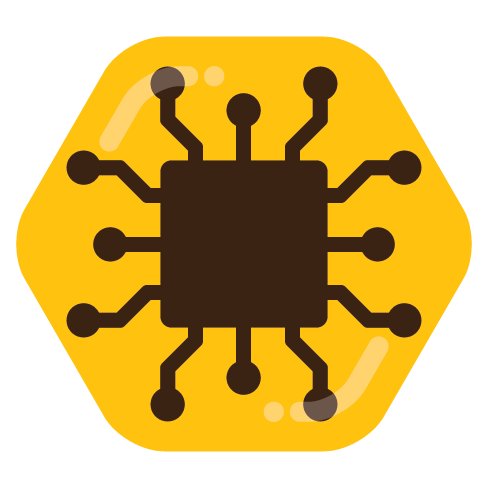
It might be because I live in the UK.
The internet I use is permanently stuck in “use phone carrier as backup” mode and we don’t have ipv6 because of that.
Data for me also seems stuck in ipv4.
Autistic queer trans²humanist and anarchist. Big fan of dense cities, code, automation, neurodiversity, and self-organising resilient networks.
Pronouns: they/them, xe/xem, ze/zem
Favourite Programming Language: Rust
Alt-Account Of: @sapient_cogbag@sh.itjust.works
It might be because I live in the UK.
The internet I use is permanently stuck in “use phone carrier as backup” mode and we don’t have ipv6 because of that.
Data for me also seems stuck in ipv4.
Yes, somewhat. The problem is places still suck at adopting it, especially phone carriers, and most people are primarily connected via their phones and a lot of people even use that infrastructure as a replacement for broadband as well.
VeilID might be something you find interesting. It’s designed to solve exactly this problem by enabling most nodes to NATsmash with help for p2p stuff, and also provides a general and very strong privacy framework including torlike routing .
It was only unveiled at defcon this year though so the team behind it (Cult Of The Dead Cow) are trying to put docs in place ;p
Its completely written in rust, easily embeddable, has good content locality and is probably the cleanest, most performant, and most easily integrated into projects architecture for stuff like this that I’ve seen, as a programmer who’s into this space and familiar with things like i2p, tor, etc. I really hope this one takes off, and the quality of it means I really think it could (at least once they throw the docs together ;p)
Be real. The cost of building means they’re always going to favour the wealthy. At best right now were running public copies of the older and smaller models. Local AI will always be running behind the state of the art big proprietary models, which will always be in the hands of the richest moguls and companies in the world.
Distribution of LoRA-style fine-tuning weights means that FOSS AI systems have a long term advantage because of compounding effects. .
That is, high-quality data provided for smaller models and very small “model finetuning” weights, which is more accessible to open groups, are sufficiently accessible and modular in their improvements to a given model that the FOSS community can take and run with it to compete effectively with proprietary groups from even a single leak.
Furthermore, smaller and more efficient models which can be run on lower end hardware also avoid the need to send off potentially sensitive data to AI companies and enable the kinds of FOSS compounding effect explained above.
This doesn’t just affect people who like privacy, but also companies with data privacy requirements . - as long as the medium models are “good enough” (which I think they are ;p), the compounding effects of LoRA tuning and better data privacy properties, and further developments which already exist in research papers towards much lower weight-count models and training mechanisms capable of greater weight efficiency to induce zero-shot learning, mean local AI can compete with proprietary stuff. It’s still early days but it is absolutely doable even today with fairly low-end hardware, and it can only get better for the reasons provided.
Furthermore, “intellectual property” and copyright stuff have an absolutely massive and arguably even more powerful set of industries behind them. Trying to strengthen IP stuff against AI means that AI will only be available to those controlling these existing IP resources and it’s unending stranglehold on technology and communication and people as a whole :/
AI I think is also forcing more and more people to look and reevaluate society’s relationship with work and labour. And frankly I think that this is super important, as it enables a greater chance of more radical liberation from the existing structures of not just capitalism and it’s hierarchies but the near-mandatoriness of work as a whole (though there has already been some stuff like this around the concepts of “bullshit jobs”).
I think people should use this as an opportunity to unionise and also try and push for cooperative and democratic control of orgs ., and many other things that I CBA to list out ;3
I hope not. Not a big fan of propriety AI (local AI all the way, and I hope people leak all these models, both code and weights), but fuck copyright and fuck capitalism which makes automation seem like a bad thing when it shouldn’t be ;p nya
What the hell are people supposed to do?
Eat the rich :)
More concretely, there are a number of smaller and larger sociopolitical changes that can be fought for. On the smaller side, there’s rethinking the way our society values people and pushing for some kind of UBI, on the larger side there’s shifting to postcapitalist economics and organisation to various degrees .)
New surveillance capitalism just dropped.
those are usually the opposite of libsoc lol
This is nothing less than a brazen attempt at total control of the primary large-scale communication mechanism of humanity.
If someone attached themselves to your body transfusion-like, and used your organs, do you think they have the right to do that against your will if they would die by being detached from your body? I.E do you think the state should have the right to lock you in a cell to prevent you from detaching your transfusion tube from that person?
I get very frustrated with the people who side with the govt here because it was a 7 month foetus. Just because it is 7 months rather than 5 or 6 doesn’t suddenly mean the government should be able to coerce the use of someone’s body as an incubator against their will.
Feels like people just don’t give a shit about bodily autonomy and such :/ nya
It means we do not see content from them, and any posts they make or comments or similar won’t show up.
Nope! :)
Also it might be worth attaching this as a secondary standard, “DiscoveryPub” or something, to avoid scope creep.
In particular, I’ve figured out a way to specify sentiment/interests efficiently and combine it reliably over federation, and the data structures required to do that.
I’ve also provided some ideas for sensible defaults (automatic selection of instances, and accounting for instance load), with incremental enhancements to specificity for more advanced users ^.^, as well as a general search mechanism that can be derived from this - though for efficiency, it might be worth trying to develop some sort of probabalistic reverse index to avoid a linear scan, if we’re talking about discovering entities like users or groups where there may be very large numbers.
I hope that if people are interested they will boost the post onto Mastodon, which afaik is where the devs and ActivityPub standards people are, and try and get the ball rolling, because my focus is elsewhere right now, and the social aspects of developing things like this are much more difficult for me than the algorithmic and architectural parts ;3
I have made a post with some work I did to make getting into the federation without requiring users to understand it much easier here, by providing a sensible automatic way to select instances randomly but weighted :)
My idea is meant to allow for a spectrum from simply “pick an instance for me” using the weightings for an assumed “the user is interested primarily in general discussion”, to “search for an instance for me related to xyz topics as a search query”, to fine-tuned discovery ^.^
The weighting is always necessary to use because it allows instances to have more control over who they accept and avoid overloading smaller instances. But you can make the default UI very simple.
This is actually kind of a general activitypub thing. I might do that but that feels like I’d have to make it was more refined and go through some formal process, and I hate doing that kind of thing and find it quite difficult, especially since my attention is elsewhere now.
I kinda just want to put it out here so people with more attention and time and knowledge can push it forward or e.g. boost it onto mastodon. Though if it really goes nowhere I might do something? Idk ;p
Each instance has provided subject trees of what it’s community is meant to be like. Moreover, it has provided the terms it believes to refer to various concepts within their subject tree.
This step is where all those terms get merged together to then be used later via some kind of search algorithm, for the more sophisticated cases.
The steps are as follows.
Apply Common Interest Weighting via the Common Interest Algorithm between the user and each possible instance.
There may be a way to use Heaps or some hierarchical datastructure to sort the instances to do this more efficiently, but as long as the implementation of the Common Interest Algorithm uses BTrees and pre-calculates lexicographically ordered maps of data it can be ensured that the cost of this kind of commonality assessment only grows with the size of the tree specified by the user and the single instance to be compared, rather than all instances (for an individual instance/user comparison ^.^).
There may also be ways to compare the user against all instances at once more efficiently that I don’t know of. But the point is, we can use the Common Interest Algorithm to assign weights for each instance/group/etc. relative to each user.
We could also use some way to convert a user search query into their Common Interest Algorithm tree weights, using the list of known terms. This is for slightly more advanced terms or people perhaps searching for communities or other groups too.
Any instances/groups/communities/etc. with alignment <0
should be immediately eliminated from the list of suggested instances/groups/communities/etc. to the user.
We already have some ranking information based on how willing and able an instance is for new users, plus we have information on how aligned each instance is with this hypothetical new user - now all a fraction from 0 to 1, as we cut out instances that have a negative alignment with the user ^.^. Then I suggest we find some simple way to join those two values together. For now, I suggest simply multiplying the alignment fraction with the weights for each instance, and then use probabalistic selection to direct the user to an instance that aligns with what they want ^.^
It may also be desirable for instances to prioritise somewhat older instances with better uptime, or more trustability (e.g. using some kind of heuristic to detect bot instances or similar), and modify the weightings based on that, or eliminate some instances ^.^
For non-instance searching or discovery, we can use the alignment ranking directly as a form of search ranking :)
Redirect the user to the “final” signup page as listed in the instance metadata, along with the parameter for their desired username. Perhaps it would be worth using webfinger
to make sure the username isn’t taken on any selected instance, and automatically selecting different instances from the list until you find one without the username taken already, with a warning.
If we’re talking about discoverability of communities or similar, you just put those in order of their direct sentiment alignment rank ^.^
I don’t think you understand anarchism.